Can the Bayesian but not the frequentist “just add more observations”?
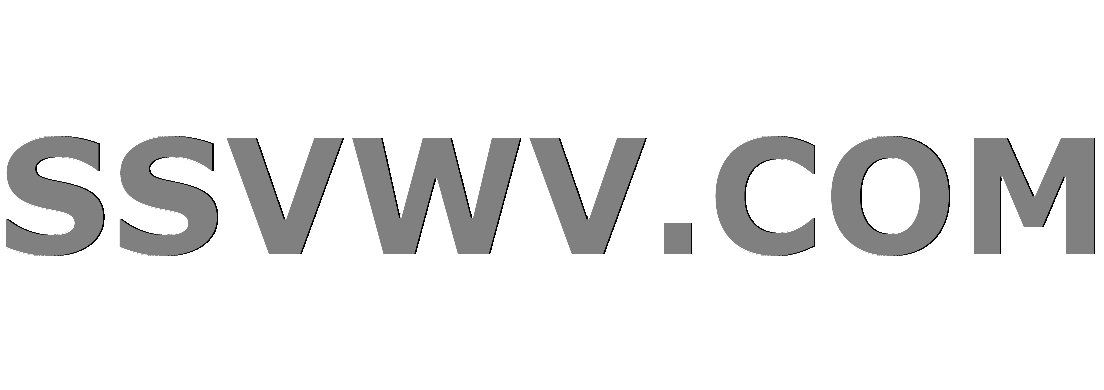
Multi tool use
Clash Royale CLAN TAG#URR8PPP
up vote
4
down vote
favorite
Since the frequentist's p-values are uniformly distributed under the null hypothesis, it is a highly problematic practice to add more and more data to your sample until you find a significant result. Assuming the null hypothesis is true, my understanding is that this will almost assuredly lead to a Type I error. This is bad scientific practice.
However, I often hear that Bayesian statistics does not suffer the same fate. Is this true?
If little evidence for the alternative hypothesis exists for some given sample size, wouldn't only stopping once there is "sufficient" evidence for the alternative hypothesis also be problematic for the Bayesian?
bayesian sampling sample frequentist sequential-analysis
add a comment |
up vote
4
down vote
favorite
Since the frequentist's p-values are uniformly distributed under the null hypothesis, it is a highly problematic practice to add more and more data to your sample until you find a significant result. Assuming the null hypothesis is true, my understanding is that this will almost assuredly lead to a Type I error. This is bad scientific practice.
However, I often hear that Bayesian statistics does not suffer the same fate. Is this true?
If little evidence for the alternative hypothesis exists for some given sample size, wouldn't only stopping once there is "sufficient" evidence for the alternative hypothesis also be problematic for the Bayesian?
bayesian sampling sample frequentist sequential-analysis
2
See sequential analysis.
– Glen_b♦
Dec 9 at 6:19
I have since come across an article by Rouder (2014; doi: 10.3758/s13423-014-0595-4). He demonstrates quite convincingly that observed posterior odds (even with optional stopping) are representative of the truth.
– NBland
Dec 10 at 4:17
add a comment |
up vote
4
down vote
favorite
up vote
4
down vote
favorite
Since the frequentist's p-values are uniformly distributed under the null hypothesis, it is a highly problematic practice to add more and more data to your sample until you find a significant result. Assuming the null hypothesis is true, my understanding is that this will almost assuredly lead to a Type I error. This is bad scientific practice.
However, I often hear that Bayesian statistics does not suffer the same fate. Is this true?
If little evidence for the alternative hypothesis exists for some given sample size, wouldn't only stopping once there is "sufficient" evidence for the alternative hypothesis also be problematic for the Bayesian?
bayesian sampling sample frequentist sequential-analysis
Since the frequentist's p-values are uniformly distributed under the null hypothesis, it is a highly problematic practice to add more and more data to your sample until you find a significant result. Assuming the null hypothesis is true, my understanding is that this will almost assuredly lead to a Type I error. This is bad scientific practice.
However, I often hear that Bayesian statistics does not suffer the same fate. Is this true?
If little evidence for the alternative hypothesis exists for some given sample size, wouldn't only stopping once there is "sufficient" evidence for the alternative hypothesis also be problematic for the Bayesian?
bayesian sampling sample frequentist sequential-analysis
bayesian sampling sample frequentist sequential-analysis
edited Dec 9 at 9:28
kjetil b halvorsen
28.2k980206
28.2k980206
asked Dec 9 at 5:45
NBland
445
445
2
See sequential analysis.
– Glen_b♦
Dec 9 at 6:19
I have since come across an article by Rouder (2014; doi: 10.3758/s13423-014-0595-4). He demonstrates quite convincingly that observed posterior odds (even with optional stopping) are representative of the truth.
– NBland
Dec 10 at 4:17
add a comment |
2
See sequential analysis.
– Glen_b♦
Dec 9 at 6:19
I have since come across an article by Rouder (2014; doi: 10.3758/s13423-014-0595-4). He demonstrates quite convincingly that observed posterior odds (even with optional stopping) are representative of the truth.
– NBland
Dec 10 at 4:17
2
2
See sequential analysis.
– Glen_b♦
Dec 9 at 6:19
See sequential analysis.
– Glen_b♦
Dec 9 at 6:19
I have since come across an article by Rouder (2014; doi: 10.3758/s13423-014-0595-4). He demonstrates quite convincingly that observed posterior odds (even with optional stopping) are representative of the truth.
– NBland
Dec 10 at 4:17
I have since come across an article by Rouder (2014; doi: 10.3758/s13423-014-0595-4). He demonstrates quite convincingly that observed posterior odds (even with optional stopping) are representative of the truth.
– NBland
Dec 10 at 4:17
add a comment |
1 Answer
1
active
oldest
votes
up vote
3
down vote
It's not that the procedure you describe (keep collecting data until you like the results) does not inflate the type 1 error, if you naively conduct repeated Bayesian analyses, it's that the brand of Bayesian that considers that there is no issue in repeatedly looking at data simply considers type 1 errors an irrelevant concept - and would likely also not favor looking at whether a credible interval excludes 0 to make a decision.
An alternative way of looking at this is to write down the likelihood based on the whole experiment - e.g. if I can never see more heads than tails, because I will keep flipping coins until I see more tails than heads, then the final outcome of the experiment clearly does not follow a binomial distribution.
Another typical way tho handle multiplicity in a Bayesian setting is to use a hierarchical model, but I have never seen a clear description of how one would do that in this context.
Let's say we do a Bayesian analysis to see whether an unbiased coin is fair (the alternate hypothesis being that the probability of heads on any given toss is not equal to 50%). There will be sequences of tosses that appear biased, even though they are due to chance. But if we stop tossing the coin when this discrepancy between observed heads and observed tails approaches what we deem significant, then that is clearly a Type I error...right? I suppose it's difficult to intuit why Bayesian statistics with optional stopping doesn't lead to false positive evidence like a frequentist approach.
– NBland
Dec 9 at 9:09
1
In case the answer was not clear: of course it does lead to a higher type I error rate, but the argument for why to ignore it is that we should not care about the type 1 error rate.
– Björn
Dec 9 at 9:13
1
Shouldn't we always care about falsely positive evidence? The Bayesian and the frequentist share the goal of good scientific practice, and Type I errors are counter to this goal.
– NBland
Dec 9 at 9:16
1
@NBland: Your last comment is very interesting and merits being stated as its own question. Maybe frequentist and bayes concerns with different aspect of inference? maybe frequency and subjective (more or less) opinion is different aspect of probability, not just diferent interpretations?
– kjetil b halvorsen
Dec 9 at 9:31
Perhaps you would rather control the false discovery rate? If you only study true null hypotheses 100% of your claimed discoveries will be false. I have a lot of sympathy for type 1 error control, but it's not the be all end all of science.
– Björn
Dec 9 at 10:15
add a comment |
Your Answer
StackExchange.ifUsing("editor", function ()
return StackExchange.using("mathjaxEditing", function ()
StackExchange.MarkdownEditor.creationCallbacks.add(function (editor, postfix)
StackExchange.mathjaxEditing.prepareWmdForMathJax(editor, postfix, [["$", "$"], ["\\(","\\)"]]);
);
);
, "mathjax-editing");
StackExchange.ready(function()
var channelOptions =
tags: "".split(" "),
id: "65"
;
initTagRenderer("".split(" "), "".split(" "), channelOptions);
StackExchange.using("externalEditor", function()
// Have to fire editor after snippets, if snippets enabled
if (StackExchange.settings.snippets.snippetsEnabled)
StackExchange.using("snippets", function()
createEditor();
);
else
createEditor();
);
function createEditor()
StackExchange.prepareEditor(
heartbeatType: 'answer',
autoActivateHeartbeat: false,
convertImagesToLinks: false,
noModals: true,
showLowRepImageUploadWarning: true,
reputationToPostImages: null,
bindNavPrevention: true,
postfix: "",
imageUploader:
brandingHtml: "Powered by u003ca class="icon-imgur-white" href="https://imgur.com/"u003eu003c/au003e",
contentPolicyHtml: "User contributions licensed under u003ca href="https://creativecommons.org/licenses/by-sa/3.0/"u003ecc by-sa 3.0 with attribution requiredu003c/au003e u003ca href="https://stackoverflow.com/legal/content-policy"u003e(content policy)u003c/au003e",
allowUrls: true
,
onDemand: true,
discardSelector: ".discard-answer"
,immediatelyShowMarkdownHelp:true
);
);
Sign up or log in
StackExchange.ready(function ()
StackExchange.helpers.onClickDraftSave('#login-link');
);
Sign up using Google
Sign up using Facebook
Sign up using Email and Password
Post as a guest
Required, but never shown
StackExchange.ready(
function ()
StackExchange.openid.initPostLogin('.new-post-login', 'https%3a%2f%2fstats.stackexchange.com%2fquestions%2f381066%2fcan-the-bayesian-but-not-the-frequentist-just-add-more-observations%23new-answer', 'question_page');
);
Post as a guest
Required, but never shown
1 Answer
1
active
oldest
votes
1 Answer
1
active
oldest
votes
active
oldest
votes
active
oldest
votes
up vote
3
down vote
It's not that the procedure you describe (keep collecting data until you like the results) does not inflate the type 1 error, if you naively conduct repeated Bayesian analyses, it's that the brand of Bayesian that considers that there is no issue in repeatedly looking at data simply considers type 1 errors an irrelevant concept - and would likely also not favor looking at whether a credible interval excludes 0 to make a decision.
An alternative way of looking at this is to write down the likelihood based on the whole experiment - e.g. if I can never see more heads than tails, because I will keep flipping coins until I see more tails than heads, then the final outcome of the experiment clearly does not follow a binomial distribution.
Another typical way tho handle multiplicity in a Bayesian setting is to use a hierarchical model, but I have never seen a clear description of how one would do that in this context.
Let's say we do a Bayesian analysis to see whether an unbiased coin is fair (the alternate hypothesis being that the probability of heads on any given toss is not equal to 50%). There will be sequences of tosses that appear biased, even though they are due to chance. But if we stop tossing the coin when this discrepancy between observed heads and observed tails approaches what we deem significant, then that is clearly a Type I error...right? I suppose it's difficult to intuit why Bayesian statistics with optional stopping doesn't lead to false positive evidence like a frequentist approach.
– NBland
Dec 9 at 9:09
1
In case the answer was not clear: of course it does lead to a higher type I error rate, but the argument for why to ignore it is that we should not care about the type 1 error rate.
– Björn
Dec 9 at 9:13
1
Shouldn't we always care about falsely positive evidence? The Bayesian and the frequentist share the goal of good scientific practice, and Type I errors are counter to this goal.
– NBland
Dec 9 at 9:16
1
@NBland: Your last comment is very interesting and merits being stated as its own question. Maybe frequentist and bayes concerns with different aspect of inference? maybe frequency and subjective (more or less) opinion is different aspect of probability, not just diferent interpretations?
– kjetil b halvorsen
Dec 9 at 9:31
Perhaps you would rather control the false discovery rate? If you only study true null hypotheses 100% of your claimed discoveries will be false. I have a lot of sympathy for type 1 error control, but it's not the be all end all of science.
– Björn
Dec 9 at 10:15
add a comment |
up vote
3
down vote
It's not that the procedure you describe (keep collecting data until you like the results) does not inflate the type 1 error, if you naively conduct repeated Bayesian analyses, it's that the brand of Bayesian that considers that there is no issue in repeatedly looking at data simply considers type 1 errors an irrelevant concept - and would likely also not favor looking at whether a credible interval excludes 0 to make a decision.
An alternative way of looking at this is to write down the likelihood based on the whole experiment - e.g. if I can never see more heads than tails, because I will keep flipping coins until I see more tails than heads, then the final outcome of the experiment clearly does not follow a binomial distribution.
Another typical way tho handle multiplicity in a Bayesian setting is to use a hierarchical model, but I have never seen a clear description of how one would do that in this context.
Let's say we do a Bayesian analysis to see whether an unbiased coin is fair (the alternate hypothesis being that the probability of heads on any given toss is not equal to 50%). There will be sequences of tosses that appear biased, even though they are due to chance. But if we stop tossing the coin when this discrepancy between observed heads and observed tails approaches what we deem significant, then that is clearly a Type I error...right? I suppose it's difficult to intuit why Bayesian statistics with optional stopping doesn't lead to false positive evidence like a frequentist approach.
– NBland
Dec 9 at 9:09
1
In case the answer was not clear: of course it does lead to a higher type I error rate, but the argument for why to ignore it is that we should not care about the type 1 error rate.
– Björn
Dec 9 at 9:13
1
Shouldn't we always care about falsely positive evidence? The Bayesian and the frequentist share the goal of good scientific practice, and Type I errors are counter to this goal.
– NBland
Dec 9 at 9:16
1
@NBland: Your last comment is very interesting and merits being stated as its own question. Maybe frequentist and bayes concerns with different aspect of inference? maybe frequency and subjective (more or less) opinion is different aspect of probability, not just diferent interpretations?
– kjetil b halvorsen
Dec 9 at 9:31
Perhaps you would rather control the false discovery rate? If you only study true null hypotheses 100% of your claimed discoveries will be false. I have a lot of sympathy for type 1 error control, but it's not the be all end all of science.
– Björn
Dec 9 at 10:15
add a comment |
up vote
3
down vote
up vote
3
down vote
It's not that the procedure you describe (keep collecting data until you like the results) does not inflate the type 1 error, if you naively conduct repeated Bayesian analyses, it's that the brand of Bayesian that considers that there is no issue in repeatedly looking at data simply considers type 1 errors an irrelevant concept - and would likely also not favor looking at whether a credible interval excludes 0 to make a decision.
An alternative way of looking at this is to write down the likelihood based on the whole experiment - e.g. if I can never see more heads than tails, because I will keep flipping coins until I see more tails than heads, then the final outcome of the experiment clearly does not follow a binomial distribution.
Another typical way tho handle multiplicity in a Bayesian setting is to use a hierarchical model, but I have never seen a clear description of how one would do that in this context.
It's not that the procedure you describe (keep collecting data until you like the results) does not inflate the type 1 error, if you naively conduct repeated Bayesian analyses, it's that the brand of Bayesian that considers that there is no issue in repeatedly looking at data simply considers type 1 errors an irrelevant concept - and would likely also not favor looking at whether a credible interval excludes 0 to make a decision.
An alternative way of looking at this is to write down the likelihood based on the whole experiment - e.g. if I can never see more heads than tails, because I will keep flipping coins until I see more tails than heads, then the final outcome of the experiment clearly does not follow a binomial distribution.
Another typical way tho handle multiplicity in a Bayesian setting is to use a hierarchical model, but I have never seen a clear description of how one would do that in this context.
answered Dec 9 at 8:49


Björn
9,5001936
9,5001936
Let's say we do a Bayesian analysis to see whether an unbiased coin is fair (the alternate hypothesis being that the probability of heads on any given toss is not equal to 50%). There will be sequences of tosses that appear biased, even though they are due to chance. But if we stop tossing the coin when this discrepancy between observed heads and observed tails approaches what we deem significant, then that is clearly a Type I error...right? I suppose it's difficult to intuit why Bayesian statistics with optional stopping doesn't lead to false positive evidence like a frequentist approach.
– NBland
Dec 9 at 9:09
1
In case the answer was not clear: of course it does lead to a higher type I error rate, but the argument for why to ignore it is that we should not care about the type 1 error rate.
– Björn
Dec 9 at 9:13
1
Shouldn't we always care about falsely positive evidence? The Bayesian and the frequentist share the goal of good scientific practice, and Type I errors are counter to this goal.
– NBland
Dec 9 at 9:16
1
@NBland: Your last comment is very interesting and merits being stated as its own question. Maybe frequentist and bayes concerns with different aspect of inference? maybe frequency and subjective (more or less) opinion is different aspect of probability, not just diferent interpretations?
– kjetil b halvorsen
Dec 9 at 9:31
Perhaps you would rather control the false discovery rate? If you only study true null hypotheses 100% of your claimed discoveries will be false. I have a lot of sympathy for type 1 error control, but it's not the be all end all of science.
– Björn
Dec 9 at 10:15
add a comment |
Let's say we do a Bayesian analysis to see whether an unbiased coin is fair (the alternate hypothesis being that the probability of heads on any given toss is not equal to 50%). There will be sequences of tosses that appear biased, even though they are due to chance. But if we stop tossing the coin when this discrepancy between observed heads and observed tails approaches what we deem significant, then that is clearly a Type I error...right? I suppose it's difficult to intuit why Bayesian statistics with optional stopping doesn't lead to false positive evidence like a frequentist approach.
– NBland
Dec 9 at 9:09
1
In case the answer was not clear: of course it does lead to a higher type I error rate, but the argument for why to ignore it is that we should not care about the type 1 error rate.
– Björn
Dec 9 at 9:13
1
Shouldn't we always care about falsely positive evidence? The Bayesian and the frequentist share the goal of good scientific practice, and Type I errors are counter to this goal.
– NBland
Dec 9 at 9:16
1
@NBland: Your last comment is very interesting and merits being stated as its own question. Maybe frequentist and bayes concerns with different aspect of inference? maybe frequency and subjective (more or less) opinion is different aspect of probability, not just diferent interpretations?
– kjetil b halvorsen
Dec 9 at 9:31
Perhaps you would rather control the false discovery rate? If you only study true null hypotheses 100% of your claimed discoveries will be false. I have a lot of sympathy for type 1 error control, but it's not the be all end all of science.
– Björn
Dec 9 at 10:15
Let's say we do a Bayesian analysis to see whether an unbiased coin is fair (the alternate hypothesis being that the probability of heads on any given toss is not equal to 50%). There will be sequences of tosses that appear biased, even though they are due to chance. But if we stop tossing the coin when this discrepancy between observed heads and observed tails approaches what we deem significant, then that is clearly a Type I error...right? I suppose it's difficult to intuit why Bayesian statistics with optional stopping doesn't lead to false positive evidence like a frequentist approach.
– NBland
Dec 9 at 9:09
Let's say we do a Bayesian analysis to see whether an unbiased coin is fair (the alternate hypothesis being that the probability of heads on any given toss is not equal to 50%). There will be sequences of tosses that appear biased, even though they are due to chance. But if we stop tossing the coin when this discrepancy between observed heads and observed tails approaches what we deem significant, then that is clearly a Type I error...right? I suppose it's difficult to intuit why Bayesian statistics with optional stopping doesn't lead to false positive evidence like a frequentist approach.
– NBland
Dec 9 at 9:09
1
1
In case the answer was not clear: of course it does lead to a higher type I error rate, but the argument for why to ignore it is that we should not care about the type 1 error rate.
– Björn
Dec 9 at 9:13
In case the answer was not clear: of course it does lead to a higher type I error rate, but the argument for why to ignore it is that we should not care about the type 1 error rate.
– Björn
Dec 9 at 9:13
1
1
Shouldn't we always care about falsely positive evidence? The Bayesian and the frequentist share the goal of good scientific practice, and Type I errors are counter to this goal.
– NBland
Dec 9 at 9:16
Shouldn't we always care about falsely positive evidence? The Bayesian and the frequentist share the goal of good scientific practice, and Type I errors are counter to this goal.
– NBland
Dec 9 at 9:16
1
1
@NBland: Your last comment is very interesting and merits being stated as its own question. Maybe frequentist and bayes concerns with different aspect of inference? maybe frequency and subjective (more or less) opinion is different aspect of probability, not just diferent interpretations?
– kjetil b halvorsen
Dec 9 at 9:31
@NBland: Your last comment is very interesting and merits being stated as its own question. Maybe frequentist and bayes concerns with different aspect of inference? maybe frequency and subjective (more or less) opinion is different aspect of probability, not just diferent interpretations?
– kjetil b halvorsen
Dec 9 at 9:31
Perhaps you would rather control the false discovery rate? If you only study true null hypotheses 100% of your claimed discoveries will be false. I have a lot of sympathy for type 1 error control, but it's not the be all end all of science.
– Björn
Dec 9 at 10:15
Perhaps you would rather control the false discovery rate? If you only study true null hypotheses 100% of your claimed discoveries will be false. I have a lot of sympathy for type 1 error control, but it's not the be all end all of science.
– Björn
Dec 9 at 10:15
add a comment |
Thanks for contributing an answer to Cross Validated!
- Please be sure to answer the question. Provide details and share your research!
But avoid …
- Asking for help, clarification, or responding to other answers.
- Making statements based on opinion; back them up with references or personal experience.
Use MathJax to format equations. MathJax reference.
To learn more, see our tips on writing great answers.
Some of your past answers have not been well-received, and you're in danger of being blocked from answering.
Please pay close attention to the following guidance:
- Please be sure to answer the question. Provide details and share your research!
But avoid …
- Asking for help, clarification, or responding to other answers.
- Making statements based on opinion; back them up with references or personal experience.
To learn more, see our tips on writing great answers.
Sign up or log in
StackExchange.ready(function ()
StackExchange.helpers.onClickDraftSave('#login-link');
);
Sign up using Google
Sign up using Facebook
Sign up using Email and Password
Post as a guest
Required, but never shown
StackExchange.ready(
function ()
StackExchange.openid.initPostLogin('.new-post-login', 'https%3a%2f%2fstats.stackexchange.com%2fquestions%2f381066%2fcan-the-bayesian-but-not-the-frequentist-just-add-more-observations%23new-answer', 'question_page');
);
Post as a guest
Required, but never shown
Sign up or log in
StackExchange.ready(function ()
StackExchange.helpers.onClickDraftSave('#login-link');
);
Sign up using Google
Sign up using Facebook
Sign up using Email and Password
Post as a guest
Required, but never shown
Sign up or log in
StackExchange.ready(function ()
StackExchange.helpers.onClickDraftSave('#login-link');
);
Sign up using Google
Sign up using Facebook
Sign up using Email and Password
Post as a guest
Required, but never shown
Sign up or log in
StackExchange.ready(function ()
StackExchange.helpers.onClickDraftSave('#login-link');
);
Sign up using Google
Sign up using Facebook
Sign up using Email and Password
Sign up using Google
Sign up using Facebook
Sign up using Email and Password
Post as a guest
Required, but never shown
Required, but never shown
Required, but never shown
Required, but never shown
Required, but never shown
Required, but never shown
Required, but never shown
Required, but never shown
Required, but never shown
2
See sequential analysis.
– Glen_b♦
Dec 9 at 6:19
I have since come across an article by Rouder (2014; doi: 10.3758/s13423-014-0595-4). He demonstrates quite convincingly that observed posterior odds (even with optional stopping) are representative of the truth.
– NBland
Dec 10 at 4:17